Next: About this document ...
Up: No Title
Previous: No Title
Subsections
Improper integrals like the ones we have been considering in class
have many applications, for example in thermodynamics and heat
transfer. In this lab we will consider the role of improper integrals
in probability, which also has many applications in science and
engineering.
The first concept we need is that of a random variable. Intuitively, a
random variable is used to measure an outcome whose value is not
certain. For example, the number of hours that a hard disk can run
before failing is a random variable because it is not the same for
every drive, even if we only consider identical drives from the same
production run. A few other examples of random variables that are important
in science, engineering, or manufacturing are given below.
- The time it takes for a packet of information to travel from one
location to another on the Internet.
- The number of miles that an automobile tire can be driven before
it fails.
- The lengths of supposedly identical bolts manufactured by a
particular production line.
- The speed of a particular gas molecule in a sample of a gas.
You may be more familiar with what are called discrete random
variables, for example the number of heads obtained in ten tosses of a
coin, which can only take a finite number of discrete values. In the
case of a discrete random variable, the probability of a single
outcome can be positive. For example, the probability that a single
flip of a coin produces tails is 50%. The situation is very different
when we consider a random variable like the number of miles a
tire can be driven before failure, which can take any value from
zero to something over 100,000 miles. Since there are an infinite
number of possible outcomes, the probability that the tire
fails at exactly some number of miles, for example 50,000 miles, is
zero. However, we would expect that the probability that the tire
would fail between 40,000 miles and 100,000 miles would not be
zero, but would be a positive number.
A random variable that can take on a continuous range of values is
called a continuous random variable. There turn out to be lots of
applications of continuous random variables in science, engineering,
and business, so a lot of effort has gone into devising mathematical
models. These mathematical models are all based on the following
definition.
Definition
We say that a random variable X is continuous if there is a function
f(x), called the probability density function, such that
- 1.
, for all x
- 2.
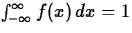
- 3.
where
represents the probability that the random variable X is
greater than or equal to a but less than or equal to b.
For example, consider the following function.
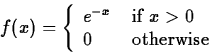
This function is non-negative, and also satisfies the second
condition, since

which is pretty easy to show. So this could be a probability density
function for a continuous random variable X.
A lot of the effort involved in modeling a random process, that is, a
process whose outcome is a random variable, is in finding a suitable
probability density function. Over the years, lots of different
functions have been proposed and used. One thing that they all have in
common, though, is that they depend on parameters. For example, the
general exponential probability density function is defined as
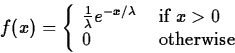
where
is a parameter that can be adjusted to get the best
fit to any particular situation. In the exercises, you will be asked
to show that only positive values of
make sense.
The process of deciding what probability density function to use and
how to determine the parameters is very complicated and can involve
very sophisticated mathematics. However, in the simple approach we are
taking here, the problem of determining the parameter value(s) often
depends on quantities that can be determined experimentally, for
example by collecting data on tire failure. For our purposes, the two
most important quantities are the mean,
and the standard
deviation
. The mean is defined by
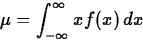
and the standard deviation is the square root of the variance, V,
which is defined by
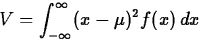
In practice, the variance V is often computed as follows,
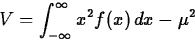
which can be easily be obtained by expanding
and writing
V as the sum of three integrals.
Probably the most important distribution is the normal distribution,
widely referred to as the bell-shaped curve. The probability density
function for a normal distribution with mean
and standard
deviation
is given by the following equation.

This distribution has a tremendous number of applications in science,
engineering, and business. The exercises provide a few simple ones.
In applications, one generally has to know in advance that the random
variable you want to model has, approximately, a certain kind of
distribution. How one would determine this is way beyond the scope of
this course, so we won't really discuss it. On the other hand, once
you know, for example, that your random variable has a normal
distribution you only need the values of the mean and the standard
deviation to be able to model it. The exponential distribution is even
simpler, since it only has one parameter, and you only need to know
the mean of your random variable to use this distribution to model it.
- 1.
- Show that the probability density function given for the
exponential distribution,
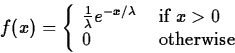
satisfies the condition
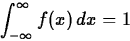
as long as
is a positive number. What would happen if
was negative?
- 2.
- Show that the mean and the standard deviation of the exponential
distribution are both equal to
. - 3.
- The amount of raw sugar that a sugar refinery can process in one
day can be modeled as an esponential distribution with a mean of 4
tons. What is the probability that the refinery will process more than
4 tons in a single day?
- 4.
- The time between accidents for all fatal accidents on
scheduled American domestic passenge airplane flights for the period
1948-1961 were found to follow an exponential distribution, with a
mean of approximately 44 days.
- (a)
- If one of those accidents occurred on July 1, find the
probability that another one occurred in that same (31 day) month.
- (b)
- Find the variance of the time between accidents.
- 5.
- Under average driving conditions, the life lengths of a certain
brand of automobile tires follow an exponential distribution with a
mean of 40,000 miles. Find the probabilities that one of these
tires, bought today, would last the following numbers of miles.
- (a)
- Over 30,000 miles.
- (b)
- Over 30,000 miles, but less than 50,000 miles.
- 6.
- The variance is defined by the following equation from the
background.
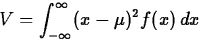
Show that the variance is also given by the following equation.
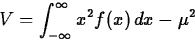
(Hint - this might best be done with paper and pencil. Part of knowing
how to use Maple is knowing when not to use it.)
- 7.
- The median m of a random variable X is the value of the random
variable such that
. If X has an exponential
distribution with mean
, find the median m.
- 8.
- The weekly amount spent for maintainence and repairs in a
certain company has an approximately normal distribution with a mean
of $400 and a standard deviation of $20. If $450 is budgeted to
cover repairs for next week, what is the probability that the actual
costs will exceed the budgeted amount?
- 9.
- Sick-leave time used by employees of a firm in the course of one
month has approximately a normal distribution, with a mean of 200
hours and a variance of 400 hours.
- (a)
- Find the probability that the total sick leave for the next
month will be less than 150 hours.
- (b)
- In planning schedules for next month, how much time should be
budgeted for sick leave if that amount is to be exceeded with a
probability of only 0.10?
Next: About this document ...
Up: No Title
Previous: No Title
William W. Farr
1/19/1999